Building Trust in AI/ML: The Key to Unlocking its Potential in Manufacturing
Manufacturers are adopting artificial intelligence (AI)/machine learning (ML) to increase efficiencies and cut costs. Using AI/ML to monitor and analyze plant operations reveals production chokepoints, potential equipment failures, and areas where automation can streamline operations. Thanks to OpenAI & ChatGPT we are also seeing a new breed of generative AI applications. These technologies will certainly have an impact on many aspects of manufacturing, but most of that impact will be in improving existing processes.
Analyzing operational information to improve performance is nothing new. Manufacturers have been applying data to address the same problems for decades; to make products better and faster while reducing costs. Proven algorithms to analyze plant operations have been in place for some time, looking for correlations that suggest causation and provide predictive insight. What has changed is the data processing technology and improved software to build applications faster with a more intuitive User Experience. Today, computers are more powerful and less expensive, making it easier to build better, more complex algorithms. We have come a long way from running analyses on mainframes using punch cards, but the fundamental problems are the same.
When applying data to assess manufacturing processes, change has been evolutionary more than it’s been revolutionary. Harnessing AI/ML is the next phase in the evolution of manufacturing data tools. In most cases, the true value is democratizing algorithms that have been used for 40+ years.
AI Success Hinges on Trust
In recent years, industrial customers were faced with a choice of “black box” algorithms; data is the input, and answers are the output. How the algorithm generates those answers should not be a concern and the message to the customer is “don’t worry the algorithm will take care of it”. However, we need a white-box approach that provides more transparency & trust into the underlying AI/ML processes.
Incorporating AI/ML means merging reliable operational data with underlying algorithms to drive better operational outcomes. Whether using linear or non-linear algorithms for predictive analytics to optimize quality or using generative AI to find and assemble information quicker, these algorithms will drive decision-making on the factory floor, so it’s essential that AI/ML-generated analytics help customers get to the “why” as well as “what” to do, with the underlying trust of “how” the insights were generated.
Creating Trustworthy AI Models
Several elements come into play when building trustworthy AI models for operational analytics:
First is the algorithm model. The algorithm needs to generate results you can trust and validate, so you need insight into how the algorithm is built. When it comes to analytics, correlation doesn’t necessarily indicate causation, so you need transparency into the algorithm, as well as how the model was built, to know you can trust the model.
Second, is the algorithm output. When you execute the model in real-time/runtime, you need to ensure transparency and reliability in the output. That includes sufficient transparency to identify reliability issues, such as if the model drifts.
Third, we can’t forget the data set used to train the model. For process data models, we must know the data is accurate. It is also important to understand what kind of data the model algorithm needs. In some cases, the models need data that is filtered and contextualized. In generative AI models, a trustworthy training data set is crucial for accurate results.
And finally, we need to trust that users are using the models in a correct way. This will require user training and a well-designed User Experience. Generative AI, like ChatGPT, is a good example. Successful results are not only based on the training data set, but also on the ability of the user to successfully prompt the AI engine. Also, understanding that generative AI knows nothing about your process and while it will produce a very coherent and mostly accurate document, trust still requires validation & human input. It’s just predicting the most likely words, it doesn’t know the impact of opening a valve.
Having visibility into the AI model is essential. In a perfect world, you can rely on an AI/ML-powered algorithm to predict physical outcomes, such as product purity. To trust that insight, you also must know when the model has shifted, and the results are no longer reliable.
AI Still Needs Human-in-the-Loop
While AI/ML has an increased role in powering plant automation, it’s still far from a “set-it-and-forget-it” technology. There is an ongoing need for the human-in-the-loop. People still need to assess the analytics and make critical decisions. In many cases, AI/ML can help solve the lack of resources problem, not by eliminating the need for people, but rather making people better at their job. In the case of plant operations, the technologies can onboard people faster and make every operator as good as the best operator. In this case, the entire operation gets better, faster.
Autopilot in the airplane industry is a good example. When planes are running on autopilot, you still have a pilot in place and pilots are still highly trained. The goal of the automation isn’t to replace the operators of “pilots” but to automate routine processes and give pilots the time to handle other critical tasks. Using AI/ML to power routine processes frees operators for more critical tasks.
There is no question that AI/ML will find an increasing role in manufacturing, but don’t expect dramatic changes overnight. It will allow plants to do more with what they have by using predictive analytics to improve product quality, using generative AI to develop applications faster, automating processes to optimize supply chain management, and even using digital twins to simulate changes to factory operations. However, AI/ML will continue to rely on a solid foundation of reliable operational data and proven algorithms. It will help make what’s already working even better.
Kevin Jones serves as the Director of Sales and Marketing for dataPARC. Kevin has been with the company since 2001 and has over 22 years of experience in process industries and using data to drive decisions. Kevin holds an undergraduate degree in Chemical Engineering from University of Idaho. dataPARC is a leading provider of industrial analytics and data visualization tools for process optimization and decision support.
Comments (0)
This post does not have any comments. Be the first to leave a comment below.
Featured Product
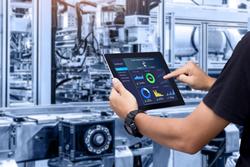