4 tips for developing predictive analytics in manufacturing
Supply cost fluctuations, constant changes in consumer behavior, and fierce competition make the manufacturing business more challenging than ever. Fortunately, the emergence of predictive analytics in manufacturing helps enterprises make data-driven decisions and stay ahead of the competition using more precise forecasting.
No wonder a growing number of manufacturers are incorporating predictive analytics into their technology stacks. According to IMARC Group’s Manufacturing Predictive Analytics Market Forecast 2022-2027, predictive analytics in manufacturing is expected to reach $2.9bn by 2027, exhibiting a CAGR of 21.2% during 2022-2027.
Despite all the benefits of predictive analytics for manufacturing, it is worth remembering that adopting it is a very demanding and challenging task. This article provides several professional recommendations to help a manufacturer ensure the success of its predictive analytics project.
Implementing in-product analytics
First, we recommend decision-makers consider enhancing their predictive analytics solution with in-built product analytics. By studying user behavior and exploring how users interact with the solution, a manufacturer can make predictive analytics more efficient and improve the user experience, leading to an increased adoption rate across an enterprise.
Here are some examples of how in-built product analytics can work in practice:
User tracking
Developers can enable automatic event tracking to understand how users interact with the predictive analytics solution. Later, developers can use this data to identify tricky or problematic parts of the user journey. With this information, developers can improve user experience to make the interaction with the software smoother and more comfortable.
A/B testing
With the help of A/B testing, developers can test new hypotheses and thus understand what software improvements to make to enhance predictive analytics. For example, developers can test new features and ensure they are relevant to employees.
KPI monitoring
Developers can customize certain KPIs, such as user engagement or login rates, to better understand whether employees are satisfied with the predictive analytics solution. Then, manufacturers can choose and track any other metrics depending on users’ behavior.
User segmentation
If dozens or hundreds of users utilize the solution, a manufacturer can segment those people into groups based on region, device, or behavior. Then, developers can tailor the predictive analytics software to each of these groups, which can also contribute to user adoption.
Validating data quality
A predictive analytics solution is only effective with relevant data that fuels it continuously. Therefore, data quality is one of the critical factors determining whether predictive analytics brings real business value.
Here are some practices to help manufacturers ensure the highest quality of their data.
Automated data validation
With the correct functionality, a predictive analytics solution can automatically validate the quality of the data. This way, employees can streamline the data validation process without doing anything manually.
Data cleansing
Given that errors and inconsistencies in data can disrupt a predictive analytics model, they should be addressed in advance, and here, data cleansing may come in handy. By equipping predictive analytics with the right in-built tools, software developers can help a manufacturer mitigate the following issues:
- Irrelevant data
- Duplicated data
- Structural errors
- Missing values
Data profiling
With the help of data profiling tools, developers can classify information based on metadata such as authorship, file sizes, and creation time. Now, any information can be found by specific keywords, significantly improving data search; this advantage can be especially relevant when working with big data.
Enhancing data security
According to IBM’s report Cost of a Data Breach 2022, an average data breach in the US costs $9.44m. Considering that predictive analytics processes vast amounts of data, we recommend that manufacturers pay special attention to the cyber security of their analytics solutions.
Here are some tips to help ensure the security of predictive analytics data.
Role-based access control (RBAC)
Developers can build the RBAC mechanism in the solution to differentiate access to data depending on the user roles and privileges. Thus, employees can access only that amount of information necessary for their work, which helps manufacturers reduce the surface of potential cyber-attacks and avoid critical data losses.
Dynamic data masking
Developers can also implement a dynamic data masking mechanism that hides sensitive data from unprivileged users. Therefore, those users won't access and view data. This measure will help to reduce the cybercrime risk.
End-to-end data encryption
End-to-end data encryption helps ensure the confidentiality of communication by transforming data (text, pictures, documents) into an unreadable format. With this mechanism built into predictive analytics, a manufacturer can ensure more secure data exchange between colleagues.
Ensuring data compliance
Data compliance should also be a top priority when it comes to the adoption of predictive analytics. On the one hand, data compliance helps automate and optimize data management. On the other hand, it contributes to data security.
Each enterprise can approach data compliance differently, so it is hard to give one-size-fits-all advice. However, in any scenario, we recommend building predictive analytics software based on the requirements of ISO standards.
Also, we recommend conducting a data audit to check if the solution adheres to particular legal requirements. For example, developers can audit predictive analytics software to determine if it complies with GDPR, HIPAA, or other regulations.
Final thoughts
Today, running a manufacturing business has become more challenging than ever. Manufacturers should adapt to constantly changing market conditions, which is only possible using the right digital technology, including predictive analytics.
The latter helps to make more intelligent business decisions in the future based on the results of past events. However, despite this obvious business advantage, implementing predictive analytics takes time and effort.
Organizations must achieve a high user adoption level while ensuring data quality, security, and compliance. Fortunately, there are time-tested practices, such as data profiling and cleansing, that can help manufacturers mitigate predictive analytics challenges.
Content is provided by at Itransition, software development company headquartered in Denver, Colorado. With more than 20 years of experience, it delivers a full spectrum of software consulting and development services, handling projects of any scale for SMBs and Fortune 500 companies from various sectors.
Comments (0)
This post does not have any comments. Be the first to leave a comment below.
Featured Product
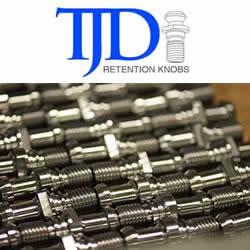