How factories Can Build Capacity and Improve Manufacturing Processes with ML
.jpg)
One of the most common problems for factories is not having sufficient production capacity to meet demand. Factories often believe they could significantly increase their production capacity if they had the facilities to do so.
Without making wholesale production changes, factories can improve process efficiency with machine learning (ML). ML can increase production capacity by up to 20% while lowering material consumption rates by 4%.
Almost all manufacturers also have the potential to integrate ML into their operations to gain predictive insights. Whether it is ensuring that the supply chain is operating efficiently or fine-tuning equipment settings to optimize a machine’s lifetime, ML can optimize processes and consequently build capacity.
Let’s look at some of the critical areas where capacity can be built with the help of machine learning.
Machine Lifetime
ML allows a manager to monitor the performance of machines, tell how many cycles they’ve gone through, and how the output of the product has changed over time. ML also enables you to predict at what point a machine needs to be replaced.
Beyond just predicting when maintenance is necessary, ML can be applied to correct process errors and extend machine lifetime. A steel factory provides an excellent example: if the pre-heat temperature of a furnace was 100 degrees too hot in the factory, this could suggest that operators had been trying to burn off the rust from the steel. Having seen the analytics from ML, a manager would then know they could resolve this problem by raising the temperature of the initial acid wash instead of the furnace. This simple adjustment could add years to the furnace’s lifetime and save the factory the enormous cost of replacing it prematurely.
Quality Inspection
The goal for manufacturers, along with increasing capacity, is ensuring that the overall quality of their products maintains a high standard. Unfortunately, it’s often not possible to inspect 100% of a factory’s products manually, but there are automated solutions that increase quality inspection capacity.
For instance, automated computer vision can increase inspection capabilities from a very low percentage when conducting a manual review of products to a 100% inspection rate. Machine learning can analyze the data itself, so it is easy to determine the quality of the output. In terms of video inspection, convolutional neural networks or other ML methods can analyze video frames on a pixel-by-pixel basis, to determine if there are sudden discolorations, instances of rust, or dimensional tolerance changes which push a product out of spec.
Utilization of Equipment
When manufacturing a product, there are so many processes that go into making just one finished part. Each process is often dependent on another in terms of how the finished part will look - the settings for one stage on the production line could directly influence the success of another step. If the data from multiple production processes can be gathered, machine learning can collate this data to conduct principal component analysis.
This gives the manufacturers an overview of machine performance and lets them know whether they can make adjustments to improve the operating capacity during the other stages of production. ML keeps factory managers from relying too heavily on institutional knowledge to make decisions on the settings of the machinery at each stage.
Labor Capacity
It’s a perpetual issue for a company that they cannot hire enough workers for the tasks they want to complete because people are the most expensive resource. With ML, the productivity of each worker can be monitored, and training can be targeted for those who need it most. For example, it’s possible to assess how many parts a worker has produced per hour in a Printed Circuit Boards (PCB) assembly line and measure this against their colleagues.
The predictive capabilities of ML can be used to determine how long a worker’s tenure at the factory is likely to be and in what stations they may be best utilized based on their employment history and skills. However, this is a slightly more theoretical application of machine learning.
ML is key to enabling predictive maintenance of machinery by identifying, monitoring, and analyzing variables during the manufacturing process. This alerts operators when a system failure might be imminent, thus avoiding costly downtime, which can prevent a factory from building capacity. Methods such as visual inspection have typically been highly manual, time-consuming, and prone to errors. Computer vision, with ML incorporated, allows manufacturers to transform quality control by automatically detecting product defects.
The categories listed above represent just a few examples of how ML can enhance manufacturing processes, but as the technology evolves, more avenues will open up to be explored.
Comments (0)
This post does not have any comments. Be the first to leave a comment below.
Featured Product
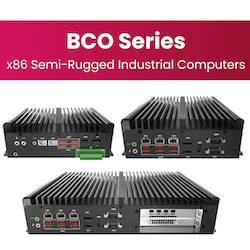