How to Find the Right Balance of Data for Your Industrial AI System
To understand the full potential of an IAI system, it’s important to look at what data, assumptions, rules, and shortcuts feed into the system during its training, testing, development, and deployment stages.
Understanding the inner workings of your Industrial Artificial Intelligence (IAI) system is crucial if you want it to add measurable value to your manufacturing operations. In this blog, we will dig into one important aspect of every AI — the inputs, a.k.a. your data. Including the right type and the right amount of data is key to success when it comes to AI.
To understand the full potential of an IAI system, it's important to look at what data, assumptions, rules, and shortcuts feed into the system during its training, testing, development, and deployment stages. You won't be able to know or anticipate everything that goes into AI from your system, but understanding these things (to the extent possible) can give you a better understanding and more realistic expectations of how your AI will behave.
What Are the Limits and Assets of My Data?
This is your classic "garbage in, garbage out" problem. Not all data is created equal, and bad data will result in bad decisions from your IAI tool. Be sure that the training data you're feeding into the system is relevant, accurate, and connected to the task at hand. It needs to have meaningful relationships to the problem you want to solve.
If you're using data that doesn't match up with real-world conditions, you're in for trouble. Look for these red flags:
Over-simplified simulations: If the data is based on simulations that don't reflect the complexity of your actual manufacturing environment, your AI might be misled.
Cherry-picked examples: Watch out for systems that only work in a narrow range of ideal situations. (AI works great on these exact three cases … just don't ask about the others.)
Excessive cleaning or smoothing: If the data has been "cleaned up" too much, it might not show the full range of variability you see on the shop floor.
Is My Data Adequate for My Use Case?
Industrial AI holds tremendous potential for revolutionizing various sectors by automating complex processes and providing actionable insights. However, the journey to effectively implementing IAI systems holds many challenges. When assessing the adequacy of your data, ask two key questions:
Am I connecting my IAI to all the relevant sources of information? It's crucial that the IAI has access to all pertinent data. This might include data from different machines, shifts, or even departments. If important data is missing or scattered across different systems, the AI may be making decisions based on incomplete information.
Does the data represent the full scope of my use case? This can be deceptively challenging to investigate. You should ensure that your data covers all the scenarios your IAI will need to handle. If the data doesn't capture enough variation, or doesn't include rare events (e.g., machine breakdowns), the system might not be reliable when those events occur. It's important to outline any gaps in the data and figure out how to monitor the AI in those situations.
Let's set one thing straight: More data isn't always better. Bigger datasets don't automatically mean better insights. What's important is the quality and relevance of the data. Too much irrelevant data just muddies the waters, while the right, focused data will help the AI system make better, more informed decisions.
Think of it this way: More data is like more bricks for building a house, but it's the quality of those bricks that determines how strong the house is. If you have the wrong kind of bricks — or too few — you won't have a solid structure. The same goes for your AI.
Common Data Pitfalls to Watch Out For
Incomplete data: Missing key data points? This can lead to unreliable predictions.
Too few representative examples: If you don't have enough examples of what you expect to see in your operation, the AI might not learn the correct patterns.
Too little variation: If your data lacks diversity, the AI may not be able to make decisions that apply to different operational conditions.
Large gaps in data: Missing data between certain states or conditions could cause the system to behave unpredictably.
Final Thoughts: Making Sure It's the Right Fit
As a small to medium-sized manufacturer, integrating IAI into your operations can offer great benefits, but it's important to approach it thoughtfully. You don't need to be an AI expert to understand the basics of how it works for your business — just follow these steps:
Ask about your data. Make sure it's relevant, high-quality, and representative of real-world scenarios.
Check for bias or imbalances. If your data doesn't accurately reflect your operation, your AI will produce flawed results.
By focusing on quality data, understanding what the AI is doing with that data, and setting clear expectations for what the tool will deliver, you'll be in a better position to make informed decisions and get the most out of your IAI investments.
Ultimately, the successful deployment of an IAI system hinges on a balanced approach to data collection, preprocessing, and evaluation. By systematically addressing the key elements of your IAI, you can make informed decisions about the value and feasibility of investing in these systems. Be sure to check out other blogs in this series including Simple Questions for Assessing Industrial Artificial Intelligence Applications.
If you have questions about Industrial AI or other technologies, contact your local MEP Center. Local experts can discuss available technologies and help you assess what technologies will align with your individual business needs.
Featured Product
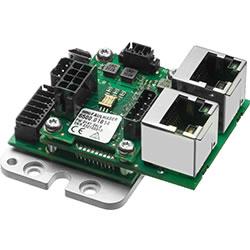