By mirroring physical assets as digital systems, manufacturers create products better and cheaper than ever before and, by bridging the physical and digital worlds, solve physical issues faster.
Transforming Manufacturing Takes Good Data Management
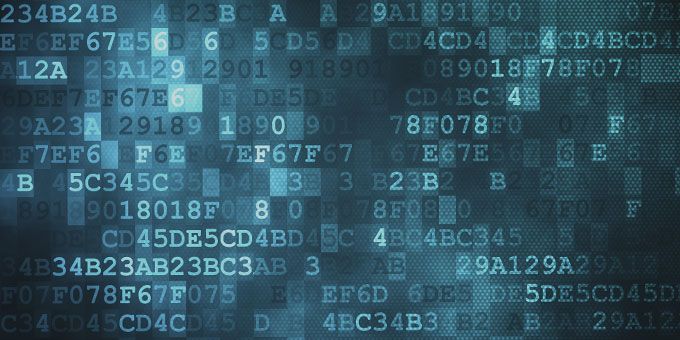
Matt Turner, Chief Strategy Officer, Media and Manufacturing | MarkLogic
Manufacturing is undergoing massive changes as it enters the 4th Industrial Revolution, which requires a fundamental shift in how we make things – from start to finish.
A real star of this revolution will be “digital twins,” the real-world digital representations of physical assets.
By mirroring physical assets as digital systems, manufacturers create products better and cheaper than ever before and, by bridging the physical and digital worlds, solve physical issues faster.
Having a digital twin offers incredible benefits. Engineering teams are better able to understand the products they build, and can improve product quality and product times. According to Gartner, by 2022, over two-thirds of companies that have implemented Internet of Things (IoT) will have deployed at least one digital twin in production. Manufacturers of IoT-connected products are the “most progressive,” Gartner says, which sets them up to successfully transform for the future.
Challenges to Digital Twins
Yet manufacturers face huge challenges in creating digital twins. Data is at the heart of digital transformation efforts and manufacturers, like many industries, have data stuck in silos, across dozens of ERP systems. That data needs to be integrated and connected for any digital transformation initiative to reach its full potential, including digital twins.
Also, traditional IT systems are not designed to support digital twins. That’s because traditional manufacturing processes involve creating long, written documents to describe systems. These document-based systems reside in emails, slides, documents or spreadsheets, often within different organizations of a business.
When a manufacturer has to change one facet of one part of a whole product, all of this information needs to be readily accessible so that engineers can quickly see how a tiny change in one requirement impacts a whole product. This gets extremely complicated in industries like aircraft manufacturing, when engineers deal with more than six million parts, or around something less complicated like a smart phone production, in which changing a component to affect screen brightness then impacts the entire system that makes up the device.
To really carry off the promise of digital twins, organizations today need a more modern approach, and that’s where model-based system engineering (MBSE) comes in. MBSE is a model-based methodology in which a single digital model depicts and relates the components and architecture of a system, and the requirements and restrictions of a system, in order for it to perform a function or for it to be safe.
With MBSE, engineers have a digital representation of everything evolved in the systems and parts to create a product. So, if someone changes a requirement around something like screen brightness, the impact on the entire system is easily mapped out and analyzed. As such, there is no losing of the overall design intent as the design changes of individual components — unless decisions are made to change the overall design.
Data is at the Heart
Dispersed data that is inconsistent and poorly managed will hinder the ability for MBSE.
Many manufacturers suffer here. They’ve been cobbled together via numerous acquisitions, developing various names for the same parts. Data might be in hundreds of different systems. Integrating all of the data in one system is expensive and could take years.
However, new database technologies offer the flexibility to handle the size and complexity of data sets critical to MBSE and digital twin capabilities by including:
Semantic capabilities. Because manufacturers may have sub-systems and components tied up within different organizations working on a small piece of an overall system, many end up with different naming conventions and uses for a single part. For example, the same screw might be referred to by many different names and used in different systems.
This data needs to mapped together and all the needed concepts need to be related. The semantic concept of an ontology is a way to manage this data with a set of relationships and objects. It defines specific objects and how they’re used within different business units in the same organization. Using screws as an example, an ontology helps everyone understand that a specific screw is the same part even if it’s referred to by different names and works in multiple parts of a product.
Modeling data using an ontology requires semantic capabilities in which the data is expressed as RDF triples. Semantics allows for consistent querying on parts and sub-systems which eliminates their need for manually curating data. This improves efficiency and streamlines processes.
A single repository of information, viewable at any time. The ability to store data in multiple formats, linkeverything, and use bitemporal capabilities in one data platform with the proper lineage is key to a successful MBSE initiative.
Next generation database technologies enable flexibility to integrate multi-model data and link it all together. Bitemporal capabilities make it easy to store multiple versions of data, on multiple timelines. Engineers can track when an event occurred (the valid time) as well as when data was entered into the database (the system time). This allows for more complex, rich queries to better understand full system impacts and how best to repair problems with the system.
Not only are complex queries possible, manufacturers should be able to trace lineage and track data provenance to ensure quality. This enables data tracing throughout its lifecycle–its origins, the path it takes and the transformations it undergoes. This provides visibility and allows data to be trusted.
Instead of dispersed data that requires manual curation, tracking a paper trail, or finding a specific subject matter expert, data can be queried in one repository.
Better analysis. Manufacturing companies need to understand the ripple effect on systems and requirements. How does a certain part (such as specific wiring or sensors) affect other parts when there are hundreds of models of just one product? If a component is 5% cheaper if there’s a 10% weight increase, is it a worthwhilechange?
Prior to MBSE, many systems were documented on paper, which required manual curation of often outdated information. If information was stored digitally, analysts could only run limited batch analysis on a current state snap-shot. And, it would take days or weeks. Today, manufacturing companies need fast and safe data analysis to remain competitive.
Using MBSE, manufacturers can complete flexible queries and explore models in real time. This allows them to perform impact analysis and validate their products while simulating the model—which is time and cost efficient.
Achieving Success
Creating digital representations of physical assets is difficult. One of the first steps to success is having data that’s integrated and searchable and supports effective MBSE methodology and analysis.
As more manufacturers explore and carry out digital twin initiatives, they’ll look to see increased cost savings, business success and higher product integrity and safety. Tackling the tough data problems will be key to reaching these goals.
The content & opinions in this article are the author’s and do not necessarily represent the views of ManufacturingTomorrow
Comments (0)
This post does not have any comments. Be the first to leave a comment below.
Featured Product
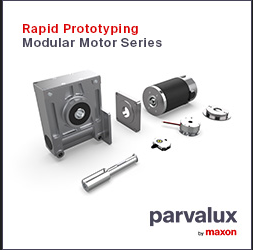