While foundational theories like Lean Supply Chain Management, Agile Supply Chain, and the Theory of Constraints have long helped businesses streamline operations, AI & ML are emerging as catalysts that can take supply chain maturity to the next level.
Unlocking the Next Era of Supply Chain Maturity: How AI and Machine Learning Drive Transformation
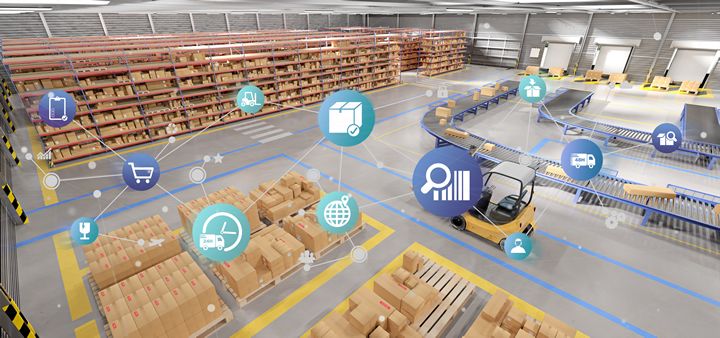
Article from | Elisa IndustrIQ
Supply chains today are complex ecosystems, where efficiency, agility, and resilience are not merely aspirational—they are essential. While foundational theories like Lean Supply Chain Management, Agile Supply Chain, and the Theory of Constraints have long helped businesses streamline operations, artificial intelligence (AI) and machine learning (ML) are emerging as catalysts that can take supply chain maturity to the next level. These technologies are not just enhancing current best practices; they are redefining what is possible in supply chain management.
Bridging Tradition with Innovation: The Role of AI and ML in Supply Chain Maturity
To understand how AI and ML can advance supply chain maturity, it is essential to revisit the principles that have driven supply chain evolution thus far.
Lean Supply Chain Management
At its core, Lean Supply Chain Management focuses on the elimination of waste and the optimisation of processes. It is about doing more with less—whether that is reducing excess inventory, cutting down on redundant activities, or improving flow efficiency.
Where AI and ML Step In: AI and ML bring predictive analytics to lean management. Machine learning algorithms can analyse vast datasets to predict demand more accurately, ensuring just-in-time inventory management without excess. AI-powered tools can identify inefficiencies that human planners might miss, constantly learning and optimising in real-time to prevent bottlenecks and reduce lead times.
The Impact: With AI, Lean principles move from static to dynamic. Continuous learning and adaptation replace periodic manual assessments, enabling companies to eliminate waste proactively before it even occurs. For example, AI can forecast supply chain disruptions caused by external factors such as weather or political instability, allowing businesses to make real-time adjustments.
Agile Supply Chain
Agility is about responsiveness—the ability to react quickly to changes in market conditions or customer demands. Traditionally, agility in supply chains has meant having flexible processes and systems that can scale or shift direction at a moment’s notice.
Where AI and ML Step In: AI and ML supercharge agility by enabling real-time decision-making. Machine learning models can simulate multiple supply chain scenarios, providing recommendations on the best course of action in minutes rather than hours. Additionally, AI-powered robotics and automation systems can quickly adapt to changing workflows, increasing speed and reducing human error.
The Impact: AI enables a truly responsive supply chain, where every component—from procurement to last-mile delivery—works in concert to adapt to fluctuations. AI can predict customer behavior and market trends, helping businesses anticipate changes before they happen, reducing stockouts or excess inventory.
Theory of Constraints
The Theory of Constraints (TOC) posits that every supply chain has one critical bottleneck that limits overall performance. The key to improving the supply chain is to focus efforts on identifying and managing this constraint.
Where AI and ML Step In: Machine learning excels at pattern recognition, making it an ideal tool for identifying hidden bottlenecks. AI can process vast amounts of data from across the supply chain to pinpoint the exact process or node that is slowing down performance. Once identified, AI-powered solutions can recommend targeted interventions, from rerouting shipments to reallocating resources.
The Impact: AI turns the reactive process of TOC into a proactive one. Instead of waiting for bottlenecks to emerge, AI continually monitors supply chain performance to identify potential constraints before they become problematic. This not only improves throughput but also enhances overall system performance.
The Maturity Model: Building a Digital Supply Chain
The integration of AI and ML into supply chains is not a plug-and-play solution. It represents a journey toward a more mature, resilient, and efficient system. Companies must evolve through several stages, each marked by increasing sophistication in their use of technology and data.
Stage 1: Foundational Automation
Many companies start their digital transformation by automating repetitive tasks like data entry, invoicing, and order tracking. While this reduces manual errors and increases efficiency, it does little to enhance decision-making capabilities.
AI Impact: Even in this early stage, AI and ML can optimise automation by learning from historical data. Predictive models can adjust reorder points or delivery schedules, ensuring that automated processes are more responsive to real-world conditions.
Stage 2: Data-Driven Insights
Once foundational automation is in place, the next step is leveraging data for better decision-making. Companies at this stage often use data analytics to gain visibility into supply chain performance, but these insights are often historical, not predictive.
AI Impact: AI turns data into actionable intelligence. Machine learning models can forecast demand, optimise inventory levels, and even predict supplier reliability based on historical performance. These insights allow businesses to shift from reactive to proactive supply chain management.
Stage 3: Predictive and Prescriptive Analytics
At this stage, supply chains move from using historical data to actively predicting future events. Companies begin to implement more sophisticated AI models that provide not only predictive analytics but also prescriptive recommendations.
AI Impact: AI-powered systems can simulate various supply chain scenarios, helping businesses choose the optimal path forward. For example, if a key supplier is delayed, AI can recommend alternative suppliers or routes, minimising disruptions. This stage is critical for companies looking to build a more resilient supply chain.
Stage 4: Autonomous Supply Chain
The pinnacle of supply chain maturity is a fully autonomous system where AI and ML make most operational decisions with little human intervention. Robotics, AI-powered transportation, and real-time data analytics work in concert to execute supply chain operations seamlessly.
AI Impact: Autonomous supply chains use AI to not only optimise existing processes but also to innovate new ways of working. For example, AI can manage entire fleets of autonomous delivery vehicles, adjusting routes in real-time based on traffic or weather conditions. With AI at the helm, supply chains can operate 24/7 without significant human oversight, driving efficiency and reducing costs.
Overcoming Challenges: AI’s Role in Risk Management
One of the most compelling applications of AI and ML in advancing supply chain maturity is in risk management. Traditional supply chain management systems often struggle to provide real-time visibility into risks. Whether it is a natural disaster disrupting logistics or a sudden spike in demand, the ability to react quickly is often hampered by a lack of timely information.
AI excels in predicting and mitigating risk. For instance, machine learning models can analyse diverse datasets—weather patterns, political events, economic trends—to predict potential disruptions. AI can then recommend proactive measures, such as shifting inventory to alternative locations or rerouting shipments to avoid affected areas.
Collaboration and Ecosystems: AI’s Role in Building Collaborative Supply Chains
Supply chains today do not exist in isolation. They are part of larger ecosystems involving multiple partners, from suppliers to logistics providers to end customers. AI facilitates better collaboration across these ecosystems by providing real-time data visibility and enabling more efficient coordination.
For example, AI can provide a shared platform where all stakeholders in the supply chain have access to the same data. This transparency helps reduce errors, improve coordination, and increase trust among partners. Furthermore, AI can optimise collaborative planning, ensuring that all parties are aligned on inventory levels, production schedules, and delivery timelines.
Conclusion: The Future is Here—AI and ML as Drivers of Supply Chain Maturity
AI and ML are not merely add-ons to existing supply chain systems; they are transformative technologies that redefine what is possible. By integrating AI and ML into supply chain operations, companies can move beyond traditional frameworks like Lean, Agile, and TOC, achieving new levels of maturity, efficiency, and resilience. The future of supply chain management is here, and it is powered by intelligent technology designed to evolve and adapt in real-time.
By embracing AI and ML, businesses can ensure they are not just keeping pace with industry changes, but leading the charge into a new era of supply chain excellence.
The content & opinions in this article are the author’s and do not necessarily represent the views of ManufacturingTomorrow
Comments (0)
This post does not have any comments. Be the first to leave a comment below.
Featured Product
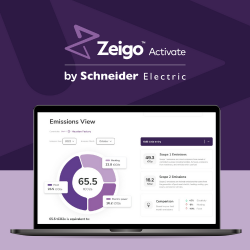