The implementation of strategic data quality capabilities can make or break a business. Many businesses suffer the consequences of risks and excess costs without ever understanding the root cause to be poor data quality or integration.
The Current State of Supply Chain Data Quality
Q&A with Chris Knerr, Former Chief Digital Officer | Syniti
Tell us about yourself and your role with Syniti.
My name is Chris Knerr, and I formerly served as the chief digital officer at Syniti, a global leader in enterprise data management software, where I lead the Data Strategy practice and drive innovation offerings globally — key strategic elements of Syniti’s strategy. I have a depth of experience in driving global business transformation, data and analytics strategy, and more, and am passionate about helping businesses develop growth strategies driven by quality data to make informed decisions that drive growth, optimize leverage and cost structure, and improve customer and quality outcomes. I’ve been a Syniti customer—at a Fortune 50 enterprise—a Syniti channel partner—at the AI start-up I co-founded—and an executive at Syniti, which gives me a unique 360 view of how we can build customer value.
How would you characterize the current state of supply chain data quality?
The implementation of strategic data quality capabilities can make or break a business. Many businesses suffer the consequences of risks and excess costs without ever understanding the root cause to be poor data quality or integration. This is particularly true in Global Supply Chains, which are incredibly and increasingly complicated. When manufacturing businesses change their operating models or execution strategies/tactics, or respond to market shocks or commercial imperatives, the effectiveness of these actions is highly data-dependent. When trusted data is available quickly and in a useful, interoperable format, it provides the foundation for intelligent decision-making. That simply isn’t the case right now for far too many firms, and bad data costs businesses dearly, both on the bottom line and—from an opportunity perspective—on the top line. This was quite evident in the last 18 months, as supply chains reeled under pandemic-induced supply and demand shocks: firms with higher data maturity and better data ‘air traffic control’ were able to respond faster, more flexibly, and more decisively to maintain supply to customers.
When the executive team needs to see reporting over multiple areas of the company, it’s incredibly difficult to present in a cohesive fashion. It also presents a limited view of customers, employees, or suppliers, as their information may be spread across multiple databases. Using data integration solutions, trusted, high-quality data can be shared across all departments, and reporting can contain real-time information based on a combination of data sources, which is supremely important to today’s global supply chains.
So, there’s lots of work to be done still to neutralize the cost of inefficient data sharing and poor data quality; it’s no wonder that 95% of executives don’t trust their data. Fortunately, there are clear software automation and organizational improvements companies can take to fix this.
What advice would you give to an organization just beginning its data transformation journey?
In the short term, it’s important to demonstrate success with smaller, measurable business cases that can be scaled up once the ROI is proven. Looking to increase language capabilities due to a geographic expansion in a particular region? That’s a great project to bring data integration and analytics in on before scaling up across the company.
In the longer term, conducting an overarching data maturity assessment is critical to sound strategy development. Understanding strategic and operational capability gaps will indicate the best mix of data operations, foundational technology, and organizational investment targets. With a data strategy in place, they are now tied to the company’s overall business strategy, which helps avoid the pitfalls of overbuilding while maximizing the opportunity gains from investments to leverage data as an asset.
Finally, a frequently overlooked area that’s critical to scaling data for value is a robust data operations approach that drives standards, business interoperability, master and meta-data management, and all the key organizational and governance capabilities that allow data to circulate smoothly throughout the organization. This is entirely indispensable to the emerging aspiration of data-as-a-utility to drive growth, cost efficiency, quality, customer experience, and compliance/risk management agendas.
What kind of goals/KPIs should businesses be setting to determine success in the short and long term?
“Pure” data programs often have a hard time ramping up and showing value unless initially linked to meaningful KPIs. That’s why I strongly recommend starting with the business metrics that are board-level and top-of-mind in the C-Suite. These can range from fundamental sales growth to EBITDA margins, to reliable supply to customers, to NPI cycle time, to excellence in the quality of products and customer service. From there, we decompose these outcome metrics into process metrics and data quality metrics that support the primary outcomes. For example, if we want to enter a new market with an existing product, is our foundational data clear about whether we have existing customers there? Do we have a way of measuring quality or outages across product lines? If we want to ensure high service levels, are we sure that our lead times and demand variabilities are accurate?
What do you think are the barriers to implementation? Are there any misconceptions you’d like to correct?
The primary challenges to implementing effective data-powered transformation programs are legacy technology debt, process debt, and data debt. Large enterprises with significant supply chain investments commonly run applications, reporting, and transactional finance within operational and technology silos. Mergers, Acquisitions, and Divestitures magnify this, as companies often acquire units with core ERP and other systems of record applications faster than they can operationally integrate them.
Data literacy, especially among senior management, also represents a core challenge. As much as we hear everywhere that “Data is an Asset,” there’s often a lack of understanding from an investment perspective that companies need to exercise the same care of data assets as they do with physical equipment, finances, or human capital.
Understanding the immediate connection of quality, trusted data to business performance and growth helps senior leadership understand why data assets are essential to core performance as well as innovation, especially in scaling AI capabilities out of the lab, and reducing friction in working across organizational boundaries with customer, suppliers, regulators, and other key stakeholders.
How can a good data strategy protect businesses from a regulatory perspective? What’s the cost of not acting?
In regulated industries, data control, compliance, and quality are paramount. Tight security, privacy, and management controls for data accuracy and completeness across the enterprise reduce non-compliance risk and associated fines and fees, which are often quite significant. Because this is an area of high complexity and continued change—especially for global enterprises that need to navigate regulatory and statutory requirements from multiple agencies—having a coherent, forward-looking strategy to respond to regulatory changes and increased oversight is also critical to maintain compliance and reduce risk.
While the penalty costs of poor compliance are generally well-understood, there are also cost, growth, and differentiation benefits that a sound data strategy covering regulatory compliance brings. For example, managing regulatory-relevant data is key from a supply chain flexibility and adaptability standpoint; without good visibility and controls, it can take months to verify whether supply chain changes like qualified ingredient substitution or moving to outsourcing are compliant for each approved market in which a company sells. And with end customers’ increasing awareness and sensitivity to privacy and data protection, firms that don’t articulate a strong posture risk brand and credibility damage, which of course is even worse in the case of actual regulatory breaches and penalties.
Regulatory compliance programs thus need not be viewed as just ‘defensive’ risk management but actually provide a great entry point for broader data transformation goals and capabilities.
The content & opinions in this article are the author’s and do not necessarily represent the views of ManufacturingTomorrow
Comments (0)
This post does not have any comments. Be the first to leave a comment below.
Featured Product
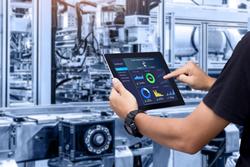