Predictive Maintenance for Industrial Equipment Using Machine Learning
In today's rapidly evolving industrial landscape, maximizing operational efficiency and minimizing downtime are paramount for businesses to stay competitive. One key strategy that has emerged as a game-changer is predictive maintenance powered by machine learning. This innovative approach utilizes advanced analytics and AI algorithms to anticipate equipment failures before they occur, enabling proactive maintenance and preventing costly downtimes.
Enhancing Industrial Efficiency and Reliability
In today's rapidly evolving industrial landscape, maximizing operational efficiency and minimizing downtime are paramount for businesses to stay competitive. One key strategy that has emerged as a game-changer is predictive maintenance powered by machine learning. This innovative approach utilizes advanced analytics and AI algorithms to anticipate equipment failures before they occur, enabling proactive maintenance and preventing costly downtimes.
Why Predictive Maintenance Matters
Traditional maintenance practices, such as scheduled maintenance or reactive repairs, often result in unnecessary downtime and increased operational costs. These approaches rely on fixed schedules or equipment breakdowns to initiate maintenance activities, leading to inefficient resource allocation and potential disruptions in production.
Predictive maintenance, on the other hand, takes a proactive approach by leveraging real-time data and machine learning algorithms to predict equipment failures well in advance. By analyzing historical performance data, sensor readings, and other relevant parameters, predictive maintenance models can identify patterns and anomalies indicative of potential issues. This foresight allows maintenance teams to intervene before failures occur, optimizing equipment performance and extending asset lifespan.
The Role of Machine Learning
Machine learning plays a central role in predictive maintenance by enabling the development of sophisticated models that can analyze vast amounts of data to identify patterns and trends. These models utilize various machine learning techniques, including supervised learning, unsupervised learning, and reinforcement learning, to extract insights from data and make accurate predictions about equipment health and performance.
Supervised learning algorithms, such as decision trees, random forests, and support vector machines, are commonly used to train predictive maintenance models using labeled historical data. These algorithms learn to recognize patterns associated with normal equipment operation and detect deviations indicative of potential failures.
Unsupervised learning techniques, such as clustering and anomaly detection, are employed to identify hidden patterns and anomalies in unlabeled data. By analyzing the relationships and similarities within the data, these algorithms can uncover emerging issues or irregularities that may signal impending failures.
Reinforcement learning, a type of machine learning where an agent learns to make decisions by interacting with its environment, is also used in predictive maintenance to optimize maintenance schedules and resource allocation. By continuously learning from feedback and adjusting maintenance strategies in real time, reinforcement learning algorithms can adapt to changing operating conditions and improve maintenance efficiency.
Benefits of Predictive Maintenance
The adoption of predictive maintenance offers numerous benefits for industrial organizations across various sectors:
Improved Equipment Reliability: By identifying potential failures in advance, predictive maintenance minimizes unexpected breakdowns and improves equipment reliability, ensuring uninterrupted operations and increased productivity.
Reduced Maintenance Costs: Predictive maintenance helps optimize maintenance schedules and resource allocation, reducing the frequency of unnecessary maintenance activities and minimizing maintenance costs.
Extended Asset Lifespan: Proactively addressing equipment issues and performing timely maintenance tasks can extend the lifespan of industrial assets, maximizing their return on investment and reducing capital expenditures.
Enhanced Safety: By identifying and addressing potential safety hazards before they escalate, predictive maintenance contributes to a safer work environment for employees and reduces the risk of accidents and injuries.
Data-Driven Insights: Predictive maintenance generates valuable insights into equipment performance and operational trends, enabling data-driven decision-making and continuous improvement initiatives.
Competitive Advantage: Organizations that adopt predictive maintenance gain a competitive advantage by optimizing their operations, minimizing downtime, and delivering superior products and services to their customers.
Implementing Predictive Maintenance
Implementing predictive maintenance requires a systematic approach and collaboration across various departments within an organization. The following steps outline the typical process of implementing predictive maintenance using machine learning:
Data Collection and Preparation: The first step in implementing predictive maintenance is to collect relevant data from industrial equipment and prepare it for analysis. This may involve integrating sensors and data collection devices into existing equipment or systems and ensuring data quality and consistency.
Data Analysis and Modeling: Once the data is collected, it is analyzed to identify patterns, trends, and anomalies that may indicate potential equipment failures. Machine learning models are then developed using historical data to predict future equipment performance and health.
Model Training and Evaluation: The predictive maintenance models are trained using labeled historical data, and their performance is evaluated using metrics such as accuracy, precision, recall, and F1-score. This process involves iteratively refining the models to improve their predictive capabilities.
Deployment and Integration: Once the models are trained and evaluated, they are deployed into production environments and integrated with existing systems and workflows. This may involve developing custom software applications or integrating predictive maintenance capabilities into existing enterprise systems.
Monitoring and Maintenance: After deployment, the predictive maintenance models are continuously monitored to ensure their effectiveness and accuracy. Regular maintenance and updates are performed to keep the models up-to-date and responsive to changing operational conditions.
Continuous Improvement: Predictive maintenance is an ongoing process that requires continuous monitoring, evaluation, and improvement. Organizations should regularly review their predictive maintenance strategies and incorporate feedback from stakeholders to drive continuous improvement and optimization.
Call to Action: Experience the Benefits of Predictive Maintenance
Ready to revolutionize your industrial operations with predictive maintenance powered by machine learning? Take the first step towards maximizing equipment reliability, minimizing downtime, and optimizing your operations by partnering with us. Visit our website today to learn more about our predictive maintenance solutions and how we can help you unlock the full potential of your industrial assets.
Contact Us:
Ready to get started? Contact our team today to learn more about our predictive maintenance solutions and schedule a consultation.
Start Your Predictive Maintenance Journey Today
Don't wait until it's too late! Join the predictive maintenance revolution and experience the benefits of proactive equipment maintenance and optimized industrial operations. Visit our website to learn more and schedule a consultation with our team.
Unlock the power of predictive maintenance with machine learning and take your industrial operations to new heights of efficiency and reliability. Contact us today to get started!
Featured Product
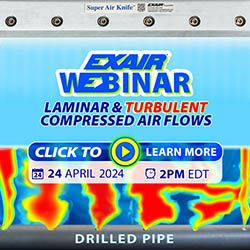
Optimizing Processes For Food and Beverage Industry Webinar
Compressed air is used in nearly all industry types within the manufacturing sector. Since it is also one of the most expensive resources in the manufacturing environment, optimizing your compressed air system for specific applications is beneficial.
This webinar will focus on applications in the Food and Beverage Industry. We'll highlight the benefits of employing an intelligent compressed air solution for tasks ranging from simple liquid spill clean-up to more complex packaging and labeling applications. We'll also look at more innovative solutions with an automation twist to perform product movements and rejections based on quality control inputs.
We will discuss several examples from the food and beverage industry to highlight these benefits and show their universal nature for other sectors.
Register for Webinar on May 14 at 2:00 pm https://exair.co/184_sweb25em