Impact of Machine Learning on Semiconductor Industry Automation
The semiconductor industry is at the heart of the modern digital revolution, enabling the rapid advancements in technology we see today. With increasing demand for high-performance and efficient semiconductor devices, manufacturers are seeking innovative solutions to enhance production processes and ensure quality. Machine learning (ML) has emerged as a transformative tool, reshaping the landscape of semiconductor industry automation.
Understanding the Role of Machine Learning in Semiconductors
Machine learning leverages algorithms to analyze vast datasets, identify patterns, and make predictions. This capability is particularly valuable in semiconductor manufacturing, where precision and efficiency are paramount. The integration of ML into automation processes helps optimize production, reduce costs, and improve the quality of chips.
Key Applications of Machine Learning in Semiconductor Automation
1. Yield Optimization
Yield refers to the number of functional chips produced per wafer. Maximizing yield is critical for profitability. ML algorithms analyze historical production data and identify factors affecting yield, such as process parameters, environmental conditions, and equipment performance. By addressing these variables, manufacturers can achieve higher yields and minimize defects.
2. Defect Detection and Classification
Defect inspection is one of the most resource-intensive processes in semiconductor manufacturing. Traditional methods often struggle with the scale and complexity of modern chip designs. ML-based image recognition systems can analyze inspection data more efficiently, identifying defects and classifying them based on severity. This accelerates the process and enhances accuracy.
3. Predictive Maintenance
Equipment downtime can significantly disrupt semiconductor production. ML models monitor equipment performance in real time, detecting anomalies and predicting potential failures before they occur. This allows manufacturers to perform maintenance proactively, reducing downtime and saving costs.
4. Process Control and Optimization
Semiconductor manufacturing involves hundreds of intricate steps, such as etching, doping, and deposition. ML algorithms analyze process data to ensure consistent quality, automatically adjusting parameters to compensate for variations. This level of control enhances production efficiency and reduces waste.
5. Chip Design Automation
The design phase of semiconductors is equally complex. ML assists engineers by simulating different layouts and predicting performance outcomes, significantly reducing the time required for chip design. This enables faster innovation cycles and improved product quality.
6. Supply Chain Optimization
The semiconductor supply chain is vast and interconnected, involving raw materials, fabrication, assembly, and distribution. ML helps predict demand, optimize inventory levels, and identify potential bottlenecks, ensuring a smooth production cycle.
Real-World Impact of ML in Semiconductors
Several industry leaders have already embraced machine learning to revolutionize their operations:
Challenges and Future Directions
While the benefits of machine learning in semiconductor automation are substantial, challenges remain:
Data Quality: ML models require vast amounts of high-quality data, which can be difficult to collect and process.
Integration Costs: Implementing ML-driven solutions can involve significant initial investments in infrastructure and training.
Algorithm Complexity: Developing and fine-tuning ML algorithms to meet the unique demands of semiconductor manufacturing is a complex task.
Despite these challenges, the future of ML in semiconductors looks promising. Innovations such as quantum computing and edge AI are expected to further enhance the capabilities of ML-driven automation. Additionally, as ML algorithms become more advanced, their application in sustainable manufacturing processes will contribute to reducing energy consumption and minimizing environmental impact.
Conclusion
Machine learning is transforming the semiconductor industry by driving automation, improving efficiency, and ensuring quality. From yield optimization and defect detection to predictive maintenance and supply chain management, ML has become an indispensable tool for semiconductor manufacturers. As the industry continues to evolve, the integration of advanced ML technologies will play a pivotal role in meeting the growing demand for high-performance chips while staying competitive in a fast-paced market.
Featured Product
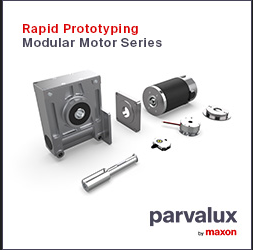
Rapid Prototyping with the Modular Motor Series
Quick to configure. Quick to build. Quick to deliver. Parvalux understands the importance of getting product in the hands of customers quickly and efficiently. The Modular Range does just that allowing customers to configure their own solution, selecting motor and gearbox, adding encoders and brakes to create a solution perfectly suited for their specific applications such as conveyor belt systems, picking systems, parcel sorting equipment, pallet shuttles and automated storage and retrieval systems (ASRS). Read our modular range guide for specifics.