With predictive analytics quality management, manufacturers may now identify and avoid quality issues before they arise by utilizing cutting-edge technology like AI and machine learning (ML), which has significantly improved productivity and profitability.
Predictive Quality Analytics in Manufacturing: How AI and Machine Learning are Transforming the Industry
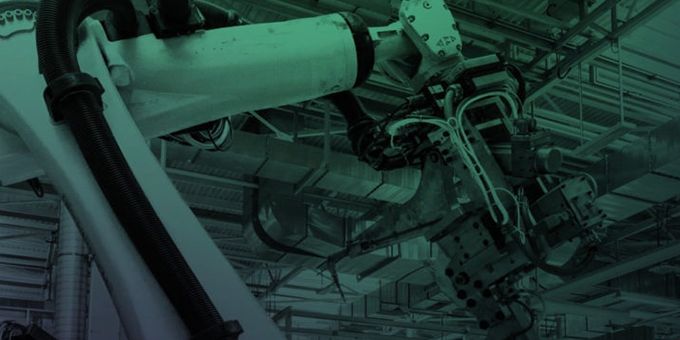
Article from | Vanti
Introduction
In recent years, manufacturing companies have been confronted with a rising challenge: how to preserve and improve the quality of their goods while simultaneously boosting efficiency and reducing costs. Predictive quality analytics can help in this situation. With predictive analytics quality management, manufacturers may now identify and avoid quality issues before they arise by utilizing cutting-edge technology like artificial intelligence (AI) and machine learning (ML), which has significantly improved productivity and profitability.
Quality Control and Predictive Quality Analytics
Manufacturers have typically depended on quality control procedures to guarantee that their goods satisfy the necessary requirements. At the conclusion of the production process, this usually entails checking a sample of the products to look for any flaws or non-conformities. This strategy, nevertheless, has a few drawbacks. For starters, it is only useful for identifying problems after they have already happened rather than preventing them from occurring at all. Inspecting each product individually can also be time-consuming, prone to manual errors, and expensive, especially for large-scale enterprises.
On the other side, predictive quality analytics makes use of data and analytics to find patterns and trends in manufacturing processes, enabling manufacturers to anticipate and stop quality problems before they arise. Analyzing data from numerous sources, including sensor readings, machine records, and quality control inspections, can be used to do this. Manufacturers can detect patterns and abnormalities in data that point to future quality problems and take preventative action by utilizing AI and ML algorithms to identify them.
Benefits of Predictive Analytics Quality in Manufacturing
Using predictive analytics tools for manufacturing has several important advantages, the primary being having the capacity to recognize and stop quality problems before they start. This can greatly lower the amount of defective goods produced and raise the standard of the entire product line, increasing consumer happiness and loyalty. Additionally, producers can avoid wasting time and money on rework and scrap by spotting and fixing quality problems early in the production process.
The capacity to increase production efficiency is another important advantage of predictive quality in manufacturing. Manufacturers can make changes and enhancements to increase productivity and save costs by locating bottlenecks and inefficiencies in the production process. Additionally, businesses can decrease downtime and boost overall equipment efficacy by leveraging data and analytics to enhance manufacturing operations by tracking KPIs like Overall Equipment Effectiveness (OEE).
Leveraging AI/ML in Predictive Quality Manufacturing
The application of artificial intelligence (AI) and machine learning (ML) techniques is one of the primary facilitators of predictive analytics quality management. With the use of these technologies, predictive algorithms can grow more precise and efficient over time as they process more data. To further boost its capabilities, predictive quaity manufacturing can also be combined with other cutting-edge technologies like the Internet of Things (IoT) and big data analytics. However, one thing to keep in mind is that data management (data collection, data preparation, etc.) is a crucial factor in the overall effectiveness and accuracy of predictive analytics. Clean production data will produce more accurate models, which is a major battle. As they say, garbage in, garbage out—even with AI.
Nowadays, modern cloud AI/ML solutions can democratize AI by turning OT or plant floor professionals into citizen data scientists by utilizing a drag and drop, intuitive UX and focusing on solving problems, instead of spending time on learning the nitty-gritties of data science practices. Armed with deep domain knowledge, these citizen AI manufacturing solutions can not only build accurate ML models for predicting quality very quickly, but also monitor and self-adjust their performance over time as their accuracy diminishes due to production data changes. Who said you need an army of data scientists to improve manufacturing quality?
If manufacturers don’t use modern predictive quality AI solutions and just use their own home-grown solutions, they must deal with the cost and complexity of implementing and maintaining an in-house Predictive analytics quality management system. This can be a significant investment for manufacturers and requires specialized expertise and resources. Additionally, organizations need to be prepared to manage the large amounts of data that are generated by predictive analytics tools for manufacturing, which can be a complex and time-consuming task.
On the other hand, a modern AI-driven predictive analytics quality management solution can be a very cost-effective option for manufacturers, as it eliminates the need for large upfront investments and specialized expertise. With the pay-as-you-go pricing model, the economics work out rather well. Additionally, cloud solutions are often provided by experienced providers who can manage the data and infrastructure, reducing the burden on manufacturers. With a citizen data scientist approach and an intuitive point-and-click interface aimed at non-technical stakeholders, it makes predictive analytics in manufacturing much more accessible. Said differently, modern AI-based predictive solutions can offer scalability, flexibility, and easy integration with other technologies, such as the Internet of Things (IoT) and big data analytics, making it easier for manufacturers to adapt to changing business needs.
Predictive Analytics Quality Management in Action: Manufacturing Use Cases
When manufacturing companies optimize their manufacturing processes using predictive technology, it creates a thorough picture of the manufacturing floor using big data and statistical analysis, enabling businesses to make wise choices and prevent expensive errors.
This kind of process optimization can be achieved in many different industries by using data analytics in quality control, including:
- producing food and drinks (for example, predicting when a batch of beer will be ready to bottle)
- chemical production (for example, identifying the optimal time for a chemical reaction)
- automobile production (for example, determining how long it takes for tires to reach their peak performance)
- aircraft production (for example, predicting when a plane will be ready for takeoff) -Producers of robots and automation (for example, identifying the optimal time to change out a worn part on an assembly line)
- producers of all kinds (for example, predicting when a machine needs maintenance)
Let’s take a closer look at the automotive industry with some additional color. A lot of automakers are integrating sensor data and machine learning algorithms to track and forecast the performance of the machinery on their assembly lines. Manufacturers can spot patterns and abnormalities that point to possible problems by examining sensor data from machinery, such as temperature, vibration, and noise levels. For instance, a machine with an odd vibration pattern may have bearing issues, which can be fixed before a failure occurs. Manufacturers may enhance the dependability and efficiency of their assembly line equipment, resulting in more output and lower costs, by adopting predictive quality to spot and address possible problems early on.
In addition to assisting in the prevention of problems with food safety in the food and beverage industry, predictive analytics quality management also helps to reduce the likelihood of food recalls and legal action. Manufacturers can find trends and anomalies in temperature, pH levels, and other parameters that could point to potential spoiling or contamination by utilizing AI and machine learning algorithms to examine sensor data. Manufacturers can spot trends and patterns in non-conformities and take preventative action to address them by evaluating data from quality control inspections. This proactive strategy assists in raising the general standard and safety of food items while lowering the likelihood of food recalls and legal action.
Conclusion
To sum it up, manufacturing leaders and OT professionals should increasingly look towards leveraging modern cloud-based, AI-powered, quality analytics management solutions to increase the quality of their goods while also boosting productivity and cutting costs. We expect a lot of innovation in this space in the coming years, and by being early adopters of predictive analytics solutions, manufacturing organizations will be more competitive with the evolution of AI—and that’s a sound business decision.
The content & opinions in this article are the author’s and do not necessarily represent the views of ManufacturingTomorrow
Comments (0)
This post does not have any comments. Be the first to leave a comment below.
Featured Product
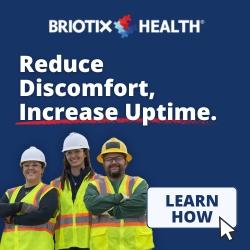