As more oil and gas companies gear up for Industry 4.0 in the wake of COVID-19 this white paper explores some of the core technologies—Digital Twins, Industrial IoT Platforms, Big Data analytics, Artificial Intelligence, and more—that accompany digital transformation.
Scaling Operational Excellence in the Oil and Gas Industry
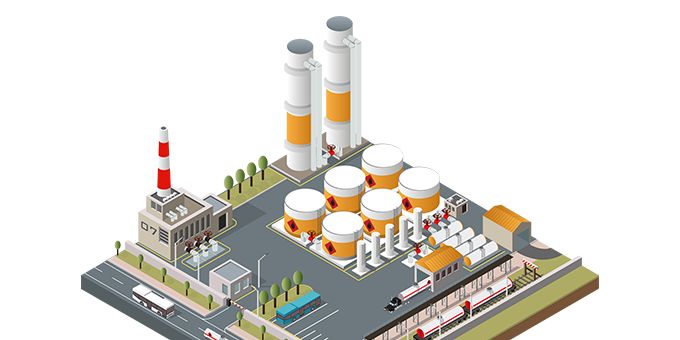
Article from | Braincube
COVID-19 was certainly the catalyst for digital transformation in the oil and gas industry, even if not for the same reasons as other industries. Pinched by diminishing demands on one end and reduced revenue on the other end, there has never been a more pressing—or necessary—time for oil and gas manufacturers to adopt Industry 4.0 technologies.
The good news is that, in some ways, oil and gas companies are well-positioned to take on digital transformation. As more oil and gas companies gear up for Industry 4.0 in the wake of COVID-19 this article explores some of the core technologies—Digital Twins, Industrial IoT Platforms, Big Data analytics, Artificial Intelligence, and more—that accompany digital transformation.
We also unpack how these tools can help oil and gas companies overcome their biggest challenges today: shrinking the skills gap, EHS, zero-emissions efforts, and more.
Primed for transformation: digital maturity
Many oil and gas companies have been collecting production data for years. Data collection is a vital part of digital transformation. After all, without data coming in from your production processes, it’s nearly impossible to analyze, understand, and learn from your processes.
The fact that the majority of oil and gas companies are already using sensors and other data-gathering equipment means that the hurdle for 4.0 is much lower than it is for other industries that are still working to collect data from their production processes. This advanced level of data collection gives oil and gas companies a strong foundational level in terms of digital maturity.
Oil and gas data collection challenges
Even though data is collected, it doesn’t mean it’s easy to use. Logging into different systems and locating the correct data is a major time suck for technical teams. So much of their time goes into locating and pulling data that they have less time to use data in their analyses, which is how improvements are identified.
There’s also a high likelihood that the data isn’t cleansed or structured into a useable format for technical teams to readily use. This is especially common if data needs to be compiled from multiple sources. There can be long delays in relaying data from off-site systems, resulting in intermittent connectivity issues.
Additionally, when data comes from different sources, teams usually need to convert it into a universally-accepted format in order to bring it together for analysis. These extra data preparation steps dampen your analytical teams’ abilities to perform analyses quickly.
These struggles go beyond just the day-to-day work for employees. Oil and gas companies are losing real value by not integrating their data systems or making data readily available. According to estimates from Ernst and Young, oil and gas companies could “potentially unlock more than $145 million of value annually by integrating key processes across the upstream oil and gas value chain with a common data model.”
These bottlenecks—decentralized data, multiple system log-ins, and uncleansed data—deteriorate your company’s overall effectiveness and trajectory for success. All this to say: data collection on its own isn’t enough.
Data cleansing and preparation with Digital Twins
With the right data-collecting hardware located in the right places, teams can gather the data they need to have a complete picture of their end-to-end production cycle. Most oil and gas companies already have these advanced sensors in place and are collecting data: the gap they need to close is making that data readily available and useful to users.
Solutions like Braincube’s Digital Twin can help close this gap. A Digital Twin is a digital replica of any process, system, or physical asset that enhances applications that serve business objectives. Using a Digital Twin enables you to merge the physical and digital worlds. Digital Twins can be built for assets, specific production lines, by end product, or for any other “real world” scenario within a production process.
Simply put, Digital Twins automate data compiling and cleansing tasks and make data instantly accessible to technical teams. A Braincube-powered Digital Twin continuously collects, cleanses, and structures data for every step of your process. This is why it is called a process Digital Twin. This type of Digital Twin accounts for every asset, process variable, lag time, and final characteristic (e.g. quality data, weather/moisture during production, etc.) using a data transformation process map.
This type of Digital Twin can be immensely valuable for oil and gas production. For example, let’s say your company has worked with Braincube to build a Digital Twin of one of your distillation columns. Using the data coming from the distillation column, teams can leverage advanced analytical tools to understand which process parameters are impacting yields or resulting in unnecessary energy consumption.
We have seen Digital Twins provide remarkable insights for oil and gas companies. One global energy company we’ve worked with already had data visualization tools, historians, and other tools for analysis, but they lacked perfect, always-up-to-date, data for analyses. Using the data they were already collecting, this energy company worked with their Braincube team to build a Digital Twin of their process by product flow.
Once the Digital Twin was built and in place, this energy company’s teams used Braincube’s apps to deep dive into Root Cause Analysis. Their initial goal was to increase the distillation yield by 20%. Using Braincube’s Apps, they identified new temperatures that led to a 36% increase in the distillation process: 16% beyond their initial target!
Self-service analytics for process optimizations and employee empowerment
According to Ernst and Young, “without skilled and trained workforces to operationalize around a common data model or to fully integrate processes across the organization, oil and gas companies will continue to leave money on the table.”
Neglecting the value of technical teams by forcing them to do tedious data gathering and cleansing may cause unnecessary attrition. Pair this with the challenges of recruiting new technical team members, plus a widening skills gap, and things look a bit bleak for oil and gas companies.
The question remains: how can companies expect to transform with a lack of technical team members?
This is where self-service analytics tools can make a major impact within the oil and gas industry. Advanced Business Intelligence (BI) Applications can play a crucial role in the digital transformation space, particularly for oil and gas companies. Self-service analytics solutions can help shrink skills gaps, empower teams to be both collaborative and autonomous, and minimize churn as employees are incentivized to learn something new.
These tools upskill citizen data scientists at your company. Citizen data scientists are employees that pair their specific process knowledge with low-code/no-code technologies, including automated Artificial Intelligence (AI), to perform advanced analyses. Empowering citizen data scientists with advanced self-service analytics streamlines the creation of data models, significantly magnifying your company’s ability to uncover solutions. Teams can spend their time fixing problems, instead of fixing data.
Sure, these models still need to be validated by your data science teams. However, implementing these advanced technologies enables citizen data scientists to fill a unique need. Self-service AI enables a process engineer (aka a citizen data scientist) to build data models and leverage their unique process knowledge. Since process engineers spend the majority of their time improving and understanding operational tasks within the factory, they can bring valuable intrinsic knowledge to data discoveries.
Braincube’s CrossRank AI is an excellent example of how technology can level up the work done by your engineers. This self-service AI is used in some of Braincube’s advanced applications. CrossRank AI was designed to parse anomalies, good and bad data, and more so that your team can get to the differences in performance, yield, or any other common KPI.
CrossRank AI is utilized in Braincube’s Comparator app. This app provides users with a straightforward, no-code template so citizen data scientists can easily dissect, compare, and uncover the difference(s) between two data populations.
For example, let’s say that one of your distillation columns starts producing fewer high-boiling-point distillates, impacting your overall yield. There were no changes to the parameters yet yield has decreased. Teams can use the Comparator App to analyze data between historical production—say, over the last three months—to production in the last 24 hours.
The app identifies each variable shared between the two data sets and reveals which variables have the largest differences between them. Shared variables with drastic differences mean higher variability. Illuminating these differences makes it easier for teams to identify the root cause of a given problem.
In this example, the Comparator App may reveal that there is an issue with the reboiler not reaching a high enough temperature to hit the necessary boiling points. Teams can focus their efforts on investigating the reboiler. This is far easier than troubleshooting from square one.
Cloud capabilities make these tools available remotely, too. Whether someone is working from their home office or a control room off-site, accessing data via the cloud means teams can continue to uncover improvements no matter where they are.
Address zero-emission efforts with Advanced AI
According to the International Energy Agency (IEA) says “that the transition to a cleaner energy system will be more difficult and expensive without the oil and gas industry.” With global energy demands expected to grow by about 25% by 2040, oil and gas companies are under immense pressure.
Compared to other industries, the oil and gas industry is in a unique position when it comes to zero-emission efforts. The challenge is not only figuring out how to make their products by using less energy. Oil and gas companies also need to increase production in order to meet the rampant energy needs expected in the future. In other words, oil and gas companies need to find a way to improve their distillation yields while simultaneously reducing consumption.
Nearly 80% of oil and gas executives plan on ramping up their AI and Machine Learning (ML) technologies in the coming years. This is a clear sign that these technologies will play a big role in shaping the future of oil and gas. We also believe these technologies will play a significant role in helping oil and gas companies meet their zero-emission goals.
Regardless of how expansive and experienced your technical teams are, AI and ML can go beyond the work of your human teams. With the ability to crunch through historical production data in minutes, AI and ML can crank through data faster than the human mind.
However, AI and ML can not be utilized to their fullest potential without your human teams. These technologies are designed to expand the capabilities of your teams, equipping them with advanced self-service analytics capabilities that do the heavy lifting. From here, teams can use their intrinsic knowledge to make sense of what the data is saying and decide what best course of action to take.
In the case of Machine Learning, computers can learn to program themselves based on data fed into a generic algorithm by users. These ML algorithms train on data delivered by data science to become smarter and more informed when giving back predictions.
Braincube’s Advanced Analysis App is a great example of how human teams can use AI to help them reach their goals. This application uses our proprietary CrossRank AI to simultaneously cross-references thousands of variables. Employees follow a template of six questions to quickly uncover the most impacting variables and optimal settings for the tested scenario.
From here, the app stack-ranks each variable in order of most to least impactful on your desired objectives. Teams can review these results, decide how to proceed, and operationalize any changes with a few clicks of a button by sending new production standards directly to operators.
Cargill, one of the world’s leading agriculture companies, used the Advanced Analysis App to minimize energy use on one of their boilers. Their goal was to optimize a boiler to use less energy while maintaining steam output, resulting in greater efficiency and cost savings.
“There was a huge opportunity to improve steam production because it is a very expensive part of our operations,” said Herber Souza, a Refinery Process Engineer at Cargill.
The Cargill team used the Advanced Analysis App to help them figure out how to decrease energy use. The app utilizes Braincube’s CrossRank AI, just like the Comparator App. Using the app, the Cargill team uncovered important operating parameters that they hadn’t considered in their initial analyses. The AI revealed that the boiler’s stability was inconsistent, making it difficult to optimize the correct conditions for sustainable boiler use.
Cargill’s process experts also utilized the Advanced Analysis App to accurately identify the best operating conditions to stabilize steam output. Once the Advanced Analysis App helped their engineering teams find process optimizations, the new recommended settings were sent directly to the operators via the Braincube Live App.
The team achieved significant cost savings in their first year using Braincube to optimize their production. They also cut their data analysis time in half, saving teams countless hours of calculations while also making improvements twice as fast: two compounding wins that continue bringing major benefits to Cargill.
“This level of impact on our production flow is transformational,” said Suelen Granado, a Chemical Engineer for Cargill.
Solving problems, identifying bottlenecks, and optimizing production with AI can be done in significantly less time and with more accurate results. Since AI leverages your past performance data to provide recommendations or discoveries, the results are rooted in what is feasible for your unique processes. These insights can be used to inform predictive models, too, enabling teams to maximize the benefits of AI.
When it comes to chasing zero-emission goals, few tools can help teams get the job done like AI.
Automated condition monitoring for improved Environment, Health, and Safety (EHS) management
The oil and gas industry is leading the charge for Environment, Health, and Safety (EHS) initiatives around the world. Much of this is due to rising global regulations around oil and gas drilling, particularly in offshore sites after the Deepwater Horizon crisis.
In 2019, the oil and gas industry saw 25 deaths in 22 separate incidents over the course of 3 billion work hours according to a report from the International Association of Oil and Gas (IOGP). While this is an improvement over previous years, it still highlights the dangers of working in the oil and gas industry—and a leading reason why EHS improvement is so high on the list for oil and gas leaders.
For example, damaged or malfunctioning equipment can cause accidents at any point in the oil and gas production cycle. Automated condition monitoring tools can help teams stay on top of factory or site conditions, hence improving safety measures for employees.
Unlike other parameters that rely on electronic sensors, corrosion relies heavily on visual inspection. In fact, visual inspection has historically been the way companies monitor mechanical corrosion. While visual sensors may not do the trick, it is possible to track key parameters—including flow rate velocity and pressure—via automated condition monitoring tools. This can help detect corrosion earlier than the human eye. A variety of sensors and probes can detect changes in these parameters, making it easier to see what is happening before something goes wrong.
In this sense, condition monitoring can feed into your predictive maintenance strategy. There are also predictive maintenance tools that make it easier to anticipate breakdowns or mechanical failures before they occur, ensuring the safety of workers.
Vibration analysis has long been used as a foundational component of condition monitoring. The Frequency Analysis node from Braincube automates vibration monitoring, making it easy to visualize and analyze high-frequency signals coming from your equipment. These visuals make it easy to see and understand what’s happening, resulting in better root cause analysis when a problem arises.
The Frequency Analysis transformation node can be paired with Braincube’s Alerts App to enhance your predictive maintenance strategies. After analyzing critical vibration conditions, teams can set alerts for when equipment trends in a similar direction. Automatic alerts are sent to key team members when unexpected conditions arise (e.g. vibrations slow down or drastically increase), enabling teams to react quickly and prevent larger issues from occurring.
Condition monitoring and preventative maintenance tools can help make your employees’ jobs easier as well as keep them safe: a win-win.
Change management to mend cultural rifts
Managing communications and collaboration between downstream, midstream, and upstream operations is incredibly complex. Significant data silos already exist between these units (as well as the different teams within them). Without a strong change management foundation, oil and gas companies run the risk of transforming as individualized silos. This means that a “new” way of working could be no different than their current way of working.
It’s estimated that 70% of digital transformation efforts fail: a daunting statistic for any executive trying to make the leap to Industry 4.0. But why are so many companies failing to transform? In our 15+ years of experience helping hundreds of manufacturers make the move to 4.0, we continuously see how underestimating the importance of change management can hinder companies from obtaining holistic transformation.
Digital transformation is about more than just buying digital resources. Buying advanced technologies will not repair cultural rifts. Adopting a collaborative, data-driven culture is often the biggest hurdle that any organization needs to overcome. Oil and gas companies are no different.
If oil and gas companies are to successfully become digital organizations, it is imperative that leadership sets the pace. Building a strong digital transformation framework enables companies to smooth over cultural gaps, utilize effective change management techniques, and emphasize cross-departmental goals that move the whole organization forward.
Effective change management also enables companies to address the skills gap plaguing the oil and gas industry. Equipping your employees with technology only goes so far if they don’t receive adequate education and training for how to use these new tools. Detailed training and technology onboarding sessions are part of a comprehensive digital transformation change management strategy.
Before COVID-19, many oil and gas companies lagged behind when it came to implementing Industry 4.0 tools, technologies, and strategies. As an industry, oil and gas still has a ways to go when it comes to advancing technical teams, repairing cultural rifts, and overhauling technology infrastructure.
However, the oil and gas industry has also made significant headway in these realms. Executives are prioritizing these initiatives and looking to solve challenges in a comprehensive way, not just by throwing money at shiny new tools.
With Industrial IoT on the rise in oil and gas, there are plenty of considerations for how to move forward. Focusing on a holistic strategy—one that merges people and technology—can help set teams on the path for short- and long-term success.
Braincube was founded in 2007 by three French engineers, Laurent Laporte (President and CEO), Sylvain Rubat du Mérac (CTO), and Hélène Olphe-Galliard. Their vision has helped thousands of manufacturers accelerate their digital transformation through data-enablement tools. Their early discovery of algorithm that could analyze and prioritize thousands of parameters and data points to revolutionize the way people in industry worked, all within minutes, was the backbone for the Braincube IIoT Platform, which has since evolved into an all-encompassing daily production tool from reporting, visualizations, and advanced analytics.
The content & opinions in this article are the author’s and do not necessarily represent the views of ManufacturingTomorrow
Comments (0)
This post does not have any comments. Be the first to leave a comment below.
Featured Product
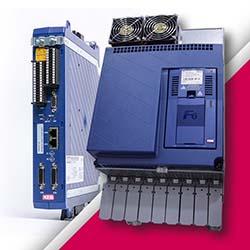