This article looks at private 5G (known as P5G) and how it supports advanced and emerging technologies, including AI cameras, enabling manufacturers to drive more functionality closer to the edge.
Private 5G Connectivity and AI Technologies Accelerate the Transformation From Automation to Autonomy
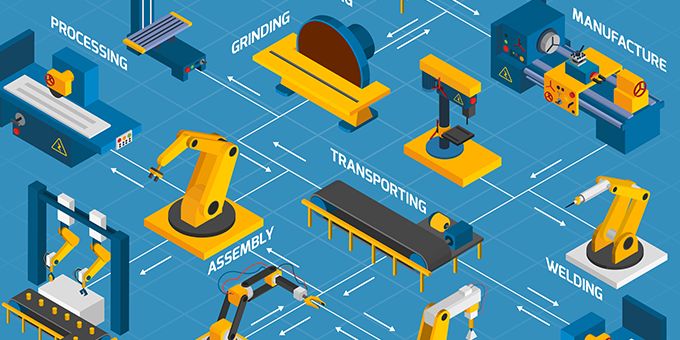
Chia-Wei Yang, Director, Edge Vision Business Center, IoT Solution and Technology Business Unit | ADLINK
Since the first mention of IoT and Industry 4.0, advances in technologies such as machine vision, artificial intelligence (AI), machine learning (ML), deep learning (DL) and wireless sensors are continuing to forge a progressive path from automation toward autonomy. However, up until recently, connectivity has come in the form of wired Industrial Ethernet, which has a huge installation cost and negates the possibility for flexible manufacturing. Wireless solutions, such as WiFi and 4G, offer that flexibility but not the speed and bandwidth, and though 4G LTE meets these criteria, its latency is lacking.
This article looks at private 5G (known as P5G) and how it supports advanced and emerging technologies, including AI cameras, enabling manufacturers to drive more functionality closer to the edge. Private 5G’s low latency is a game-changer and, together with its high bandwidth, can capture near-real-time insight into manufacturing operations. The switch from automated guided vehicles (AGVs) to autonomous mobile robots (AMRs) with swarm intelligence, for example, is just the beginning. The article covers a few use cases where 5G connected AI-enabled devices can sense their environments and interoperate with each other, making decentralized decisions. Finally, the article looks towards the near and mid-term future.
The 4 stages of IoT Development
Connectivity, storage and computing power are the foundations for enabling IoT. The first hurdle to overcome on the road to transfer legacy operations into a smart factory is that different installed machines may use different controllers such as PLC, PC and MCU, as well as a variety of machine protocols, such as Modbus, DeviceNet, CAN bus and even proprietary protocols. Many older machines even lack any communication. The second hurdle is that the machine builders may have developed proprietary source code, making it difficult for engineers to change or upgrade to maximize operations for a specific factory’s requirements. Finally, some factory managers were reluctant to allow system integrators to add, delete or modify applications for currently installed machines.
For these types of applications, companies such as ADLINK developed solutions to effectively connect the unconnected legacy machines. For example, ADLINK’s data extraction series is capable of remotely controlling and retrieving data from non-connected installations, converting essential data from devices with no output to networks. Initial IoT deployments were passive in nature, having limited computing power with embedded controllers for simple tasks. The collected data from sensors and other devices was stored in a centralized location, such as a data lake, and processed and analyzed based on Big Data architectures. Insights from the analysis were used to visualize field-side data to understand the patterns and any correlation. Many of these insights were used by machine operators for predictive maintenance to maximize machine uptime, thereby improving productivity and saving cost.
The second stage brought the factory into a more active environment with Edge devices and connectivity to share results with other Edge devices among the Industrial IoT (IIoT) network. By adding artificial intelligence (AI) into the mix, IIoT implementations moved away from presenting just the facts on what had happened, albeit in the recent past, and could automatically take action.
With the introduction of swarm intelligence, simple Edge devices can interact with each other locally. A concept found in colonies of insects, such as ants and bees, swarm intelligence is the collective interaction amongst entities. To adapt to the manufacturing environment’s dynamic evolution, the swarm of entities are self-organized, manoeuvring quickly in a coordinated fashion. Although the swarm group is limited in terms of capability and size, this low-level autonomy builds on the use of cognitive AI and ML technologies.
For IoT technology to become truly pervasive – stage 4 - latency levels need to drop to allow real-time decision making, and IoT deployments need to operate more autonomously. Private 5G is perceived as the connectivity answer, pushing more intelligence to the edge of data networks and reducing latency. At the same time, Artificial Intelligence of Things (AIoT) technology is gradually reducing the role of human decision-making in many IoT ecosystems.
Connectivity technologies of the near past
The majority of IoT implementations are currently at stage 2, using a combination of wired Industrial Ethernet with WiFi, 4G, and more recently, 4G LTE technologies as the backbone for connectivity to field-side devices. There are limitations with these wireless connectivity technologies in terms of speed and bandwidth. More importantly, latency, which is the time required for data to travel between two points, is becoming a key focus. Consider even the super-fast 4G LTE; its latency is 200ms, which is ‘real-time’ enough for some decision-making actions, but not fast enough for safety-critical decisions, such as shutting down a piece of equipment to avoid an accident.
The promise of 5G
Although public 5G roll-out raises concern in data security and might not offer consistent latency, the roll-out of enterprise-wide Private 5G (P5G) is accelerating. Private 5G boasts low latency, demonstrated by 4G’s latency of 200ms to P5G’s 1ms. P5G’s high speed and bandwidth, together with AI/ML technologies for the intelligence, enables factory operators to capture near-real-time insight into manufacturing operations - being proactive instead of reactive to events.
This applies to robots, cameras, vehicles and all edge AI applications requiring reliable, secure real-time networking to share information. Good examples of how these technologies are combined are autonomous mobile robots (AMRs) and machine vision for productivity and worker safety.
From AGVs to AMRs
The switch from AGVs to autonomous mobile robots (AMRs) with swarm intelligence, for example, is just the beginning. But what does that mean? AGVs follow a mapping track and require expensive infrastructure and additional personal safety measures. A swarm of AMRs, on the other hand, can carry out their jobs with little to no oversight by human operators. They can sense their environments and interoperate with each other, making decentralized decisions.
One of the enabling technologies for swarm autonomy, in addition to AIoT, is the second-generation Robotic Operating System (ROS 2). The open-source framework for robot software development integrates Distributed Data Service (DDS) to provide a uniform data exchange environment to share data collectively like a data river. It permits multi-robot collaboration and reliable, fault-tolerant real-time communication.
Taiwanese contract electronics manufacturer Foxconn has set up a joint venture with ADLINK, called FARobot (Foxconn ADLINK Robot), to develop ARMs. In this partnership, ADLINK provides the Eclipse Cyclone DDS software – a platform used for navigation. The AMRs use the software to share data between edge devices in real time, avoiding the cost and delay of sending data to the cloud.
Using a swarm of AMRs to carry semi-finished products to the next workstation is a typical scenario. If the total payroll needs to go to different destinations, one AMR can carry half the batch to production cell ‘A,’ another carries 30% to production cell ‘B’ and the last one takes the rest to production cell ‘C’. As the AMRs operate collaboratively and visualize field-side data using AI-enabled cameras, they know how to prioritize what percentage of the payroll goes where. The swarm has the intelligence to design and decide the queue automatically. Only combining all these technologies makes a smart factory possible, enhancing worker safety, efficiency and throughput.
SOP Compliance Monitoring
Another scenario utilizing AI machine vision is standard operating procedure (SOP) monitoring. SOPs are developed to optimize product quality and cycle time, as well as protect workers from dangers. However, human error is the primary failure factor.
Traditionally, industrial manufacturers audit SOP compliance by manual monitoring. Manual monitoring can vary among different production managers and is usually only for limited periods. Keeping track of every step performed by each operator is too time-consuming to implement through all the production lines. The workflow data is therefore incomplete, and it takes time to consolidate and then conduct analysis. This delayed response to amend incorrect procedures may lead to quality issues, lower productivity or even workplace accidents.
Taiwanese LCD panel maker, AUO, is now using AI smart cameras and behavior analysis deep learning algorithms to take care of these requirements. The solution is based on the NEON AI smart camera from ADLINK, which integrates Intel Movidius or NVIDIA Jetson AI vision processing cores with a wide range of image sensors. It comes pre-installed with an optimized operating system and software. The Edge Vision Analytics (EVA) software development kit (SDK) supports Intel OpenVINO AI and NVIDIA TensorRT, as well as field-ready application plugins, for streamlining AI vision project development time. In addition, EVA is a no-code, low-code software platform, which requires minimum programming for engineers to retrieve their AI vision analysis. In the times when everyone wants to benefit from AI but is not equipped with qualified knowledge and skills, EVA comes in handy for either manufacturers’ in-house system integrators or AI project owners to quickly conduct the proof of concept (PoC) for the AI vision applications.
These technologies enable consistent and continuously optimized SOP monitoring and assessment, enabling production managers to shift their valuable time to perform more productive tasks. Real-time analysis of AI vision also enables them to respond to incorrect procedures immediately, saving rework costs and material losses. It can also prevent operators from dangers when operating with the incorrect procedure. Comprehensive monitoring can also help identify operators who may need further training to improve cycle time. Production managers can now shift their valuable time to do more productive tasks.
The Future of Smart Manufacturing
Manufacturers are eager to make a move to a smart factory that is capable of handling mass customization. To achieve this, the conventional concept of production needs to change completely. Digital transformation will enable the smart factory to manufacture a wide variety of products without changing the production line, layout or worker allocation. Where AMRs pick up the parts and tools that are required for production and deliver them to the workstation. At the workstation, autonomous robots build the products and, once completed, are picked up and sent directly to the customer via the autonomous logistics channel. Workstyle will also likely change. For instance, workers will no longer need to enter hazardous locations and can instead use augmented reality (AR) and digital twins to visualize and monitor the operational status in real-time from the office.
While P5G-enabled IoT connectivity is the digital backbone to smart manufacturing, AI is the brain, making decisions that control the overall system. The combination of AI and IoT brings us AIoT, delivering intelligent and connected systems that are capable of self-correcting and even self-healing themselves. ADLINK’s AIoT ecosystem of alliances and partners will continue to help accelerate the deployment of AI-at-the-edge solutions for smart manufacturing – moving from automation to autonomy.
About Chia-Wei Yang
As director of ADLINK’s IoT solution and technology business unit, Mr. Chia-Wei is in charge of the company’s smart manufacturing market and business. Chia-Wei Yang has 15+ years’ experience in developing machine vision and automation innovation as well as creating value with ecosystem partners. With sophisticated knowledge in smart manufacturing as well as AI AOI applications, he ensures customers and partners have the best journey possible when increasing the efficiency of manufacturing with the architectural offerings and services. Mr. Chia-Wei Yang is in charge of ecosystem partner engagement and strategic vertical market development.
The content & opinions in this article are the author’s and do not necessarily represent the views of ManufacturingTomorrow
Comments (0)
This post does not have any comments. Be the first to leave a comment below.
Featured Product
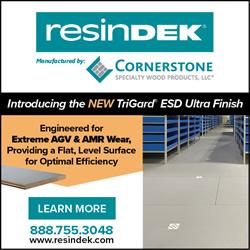