Using available data, manufacturers can identify changes or anomalies in demand, combined with accurate risk management and forecasting solutions.
Here’s How to Make the Most of Predictive Analytics
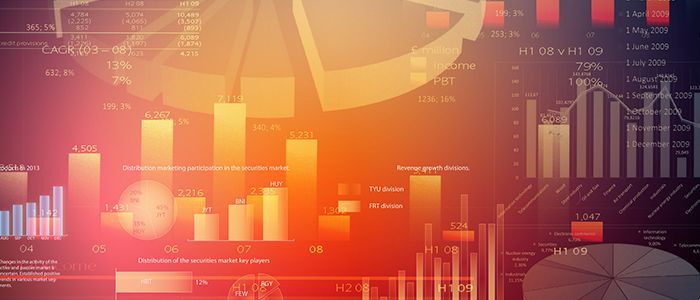
Megan Ray Nichols | Schooled By Science
The world of business is changing more and more each day, not just because of innovative technologies but also because of the new opportunities they present. Big data and advanced analytics are two excellent examples of this — things that just wouldn’t be possible without modern technology.
The massive amounts of data — collected by IoT devices — can be used to do remarkable things. Suddenly, it’s possible to create predictive profiles and make more informed decisions about future events or actions. Imagine knowing exactly how a product launch is going to play out before the item is even manufactured? That is exactly the kind of information that can be extracted and presented by big data solutions.
Predictive analytics has its place all along the supply chain, from manufacturing and development to distribution and retail. However, it’s in manufacturing where it can provide the most potential.
Perhaps Deloitte Consulting sums it up best: “With a digital supply chain supported by advanced predictive analytics coordinating three principal partners — nine countries, 40,000 individual parts and thousands of suppliers — a major manufacturer of these jets predicts it will soon be able to build one jet per day, a process that used to take months or years.”
How Predictive Analytics Are Used in Manufacturing
When you have incredibly diverse collections of data, you can scour it digitally to discern certain trends and insights. On a basic level, you can determine what elements of a product resonate with customers and which do not. This information can then be used to design and manufacture something better, a more optimized version, if you will.
This example highlights more than just one of the ways in which predictive insights can be used — it also shows how to apply incoming data. To that end, real-time and predictive data can improve operations and processes, optimize systems, find new opportunities and measure market relations.
With manufacturing, there are dozens — if not hundreds — of different components that make up an entire operation. It stretches from the equipment and machinery to the personnel, all the way back to the various design processes and forward to the managers overseeing operations. More data about everything and everyone involved provides a deeper understanding of not just what’s happening, but also what can be done to improve the bottom line.
The incoming data allows for the following to happen:
Predictive and Preventive Maintenance
Sensors embedded within machines and hardware can deliver real-time data about their current status, working conditions and even the surrounding environment. When fed into a remote monitoring system, the information can be used to detect potential problems or even upcoming maintenance requirements. Service technicians and engineers can then administer the appropriate solutions to keep the equipment operational and optimized.
This prolongs the life of equipment and boosts output by reducing the total amount of downtime that happens within a plant or facility.
More Quality Improvements Are Made Possible
Identifying problems in a manufacturing process takes time, even when there are major defects with an item or product. However, the increased troves of data can be aggregated faster and acted upon in a timelier manner. As a result, the entire manufacturing product line gets continual adjustments, sometimes even immediately upon the discovery of a problem.
It all translates to a higher-quality output with fewer delays.
Demand Forecasting Is More Accurate Than Ever
When manufacturing goods that are in high demand, the results are always hit or miss. You can have items that lose demand as you’re making them, just as you can have products that see a boon. More commonly, the overall market for certain goods wavers, which makes production difficult.
Conventional data solutions would see you adjusting based on past performance. Predictive analytics allows this to happen not just in real-time, but also in advance of a major shift. Using available data, manufacturers can identify changes or anomalies in demand, combined with accurate risk management and forecasting solutions. Together, all this provides an incredibly useful profile of how a product will fare, allowing for the appropriate development levels to be met.
How to Implement Predictive Analytics
The final part of this entire equation involves learning how to properly implement predictive analytics, especially within an existing operation.
Organizations must incorporate modern technology, such as IoT devices and sensors, to bring in more detailed data streams. Then, it’s a matter of adopting the appropriate analytics solutions, which will comb the incoming information. Raw information alone is not as useful as processed datasets as processed datasets, complete with extracted intel.
Here’s how you can make it all work:
1. Define your objectives: Decide before implementing any technologies or solutions what you would like to achieve. What is it you want your new data systems to predict?
2. Build the necessary infrastructure: To make predictive analytics possible, you will need various streams of incoming data. Sensors and devices need to be installed to capture the data you need. A network needs to be put in place to support these devices and data streams. Security solutions must be used to protect them all. Build your infrastructure around the entire network of data solutions.
3. Turn everything on: This seems almost silly, but it’s an important step. Activate your infrastructure and enable all your devices and sensors. Then, monitor them for a short term to ensure they are collecting exactly the information you need to meet your goals.
4. Choose a specific goal: With data coming in, you need to set a finish line for your initial operation. What is it you hope to achieve with your new system? At what point have you reached success? Obviously, it’s based on the objective you chose in step one.
5. Start studying the data: Once the information is coming in, you’ll need to do something with it. Whether you physically hire data scientists to parse information or you go with something more automated, you need a system in place that builds actionable intel from the incoming data streams.
6. Build your predictions: The more insightful data can then be used to build your predictions, generally through a correlation or trend. If stakeholders are involved, this also might be the ideal time to share progress with them. Are your initial predictions quantified by the available data?
7. Maintain everything: There’s a saying that goes garbage in, garbage out. It’s never been more accurate than dealing with such a massive influx of digital content and information. Be sure to maintain your data streams, keep everything organized, and verify the entire system is collecting what you need accurately.
In the end, predictive analytics is nothing more than making an educated guess — albeit with a collection of useful insights. Predictions will almost always fit into statistical models, which can be defined and repeated. More importantly, those prediction models grow stronger and more accurate over time as they are tested and amass more supporting data.
To make the most of any data analytics platform — predictive models included — consistent operations are key.
The content & opinions in this article are the author’s and do not necessarily represent the views of ManufacturingTomorrow
Comments (0)
This post does not have any comments. Be the first to leave a comment below.
Featured Product
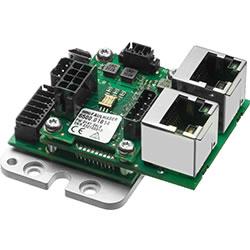