To successfully empower product and developer teams with data, iteration speed matters. Rather than having a central team determine what data can be used and who can access it, agility is essential.
Why Data Empowerment Matters
Kyle Seaman | Sentenai
Data is a core asset and differentiator across all industries, which has led to continued growth in a variety of data-driven applications. Beyond consumer-facing use cases, data-driven applications are increasingly being used within the enterprise to optimize operational productivity, improve customer service experiences and streamline business costs. In fact, some of the biggest companies in the world, like Google, Facebook and Amazon, have achieved the unprecedented scale and impact they’re famous for because of their data-driven approach to product development and customer experience.
Data-Driven Applications Require Accessibility
Tech giants aren’t the only organizations that can effectively leverage data-driven applications, however. By proactively applying objective insights into a specific business process, any organization -- no matter its size or industry -- can find success in reliably predicting customer demand or adjusting operational workflows to optimize productivity. That said, achieving sufficient data-driven application scale ultimately requires that product and developer teams have access to the data in question.
Too often, data is locked within certain departments, or it remains trapped on factory floors or in legacy database systems. To fully understand what data is available to work with and support an iterative development process, however, data must be made accessible. Taking days or weeks just to get access to data -- data that in the end may not even be useful for the current project -- significantly adds to the overhead of data-driven initiatives and can jeopardize overall company support. Furthermore, a lack of individual data empowerment can result in weakened motivation and effectiveness across product and developer teams.
Internal Analytical Hubs Offer Access and Scalability
In an effort to make data more accessible, many organizations’ product and developer teams are starting to build shadow IT analytical hubs. As nimble, standalone tools, these hubs don’t burden IT departments or risk bringing down company infrastructure due to too many parallel analysis workloads. They also allow product and developer teams to better support data-driven application development all the way from the exploration stage, to model development, to shipping.
Because product teams know any successful enterprise product will only result in large demand being placed on company infrastructure, establishing an internal analytical hub that can scale is paramount (in fact, this is precisely why IT departments are often circumvented). From the developer side, an ever-growing toolset has reduced the traditional time and resource barriers required for building analytical hubs. This allows both product and developer teams to focus on what truly matters: delivering value via their products to end-users (whether that be internal operators, various business units, or external customers and partners).
Data Agility and Nimble Prototyping are Critical
To successfully empower product and developer teams with data, iteration speed matters. Rather than having a central team determine what data can be used and who can access it, agility is essential. Most development teams today expect their infrastructure teams to support the innovative products and experiments they want to run, rather than simply abiding by whatever limited data they are told they can use to try and solve problems. In fact,GraphQL was created in direct response to this reality. Using GraphQL, product and developer teams can define what they need, while also solving core scaling and versioning challenges and reducing barriers to iterating on new ideas.
Equally critical to fostering agility is not overthinking the infrastructure and architecture required of data empowerment. Since any product and developer team’s objective is to prove value in their data by delivering impactful applications, it’s important to start prototyping as soon as possible. If teams are required to run everything through a lengthy IT approval and acquisition process, data-driven application projects will lose momentum immediately (not to mention, evaluating for a prototype versus for core infrastructure is a very different task). Also, it’s important to encourage data owners to coordinate with owners from different departments to ensure valuable data being generated is being properly socialized across the organization.
Leverage Data Empowerment to Deliver on Digital Transformation Agendas
It's no longer a question of if internal analytical hubs can be built, meaning more experiments and ideas than ever are being implemented across organizations, often in parallel. To effectively assess the validity of these experiments before over-investing and help ensure eventual success, prioritize data agility and work to improve prototyping iteration speeds. In doing so, organizations can finally realize the wealth of data they’ve already been generating as it relates to operational expertise or product market insights, and successfully deliver on digital transformation agendas by empowering their product teams to leverage one of the newest asset classes: data.
The content & opinions in this article are the author’s and do not necessarily represent the views of ManufacturingTomorrow
Comments (0)
This post does not have any comments. Be the first to leave a comment below.
Featured Product
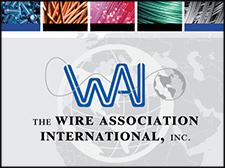